State of the art in Voice Cloning: A review
By María Noel Espinosa
Introduction
Get ready to explore the latest advances in voice cloning technology with this comprehensive review of the most recent advancements. In this blog, we will dive into the current state of voice cloning technology, including the cutting-edge research and developments in the field. We will also discuss the potential applications and ethical considerations of voice cloning, as well as the challenges that still need to be addressed to improve available implementations. Finally, we are going to explore TorToiSe: a specific tool for voice cloning. Whether you are a researcher, developer, or simply interested in the current trends in technology, this blog is for you. So, join us as we explore the fascinating world of voice cloning.
What is voice cloning?
Voice cloning refers to the process of creating a synthetic voice that is almost identical to a real human voice. Unlike speech synthesis, which generates realistic speech with predefined voices, voice cloning technology is capable of replicating a person’s unique voice, tone, and inflections.
Text: This is an example of what can be done with voice cloning and how it is different from speech synthesis.
Why is it relevant?
This technology is relevant for several reasons. First and foremost, it has the potential to revolutionize the way we interact with technology. With voice cloning, we would be able to create realistic and human-like virtual assistants, chatbots, and other speech-enabled devices. This means that we can have more natural and engaging conversations with these devices, making them easier and more pleasant to use.
Also, this technology may be used by individuals with speech impairments or disabilities. This could allow them to create synthetic voices that closely resemble their natural voices, improving their ability to communicate effectively. Additionally, voice cloning can be used to preserve the voices of people who are at risk of losing them due to medical conditions. Finally, this technology has implications for the entertainment and media industries. In particular, it will allow to create more realistic and convincing voice overs for movies, video games, and more.
In summary, voice cloning has the potential to improve the quality of life for many individuals, enhance user experience with technology, and create new possibilities in various industries.
Ethical implications surrounding voice cloning
The development of voice cloning technology raises ethical concerns around issues related to both consent and identity. Obtaining consent is an important consideration, as it may not always be clear whether individuals have given their consent for their voice to be used. Establishing clear guidelines around obtaining consent for voice cloning is necessary to ensure that individuals have the right to control how their voice is used.
Additionally, a synthetic voice can be used to impersonate individuals, and cause harm, when it is indistinguishable from a real voice. Thus, to prevent the misuse of voice cloning technology, some regulations must be established. By addressing these ethical considerations, we can ensure that the potential benefits of voice cloning technology are realized while minimizing the potential risks and harms.
State of the art
The state of the art is moving fast so it is hard to stay up to date. Following is an overview of some of the most relevant latest papers you will want to know about.
ZSE-VITS: A Zero-Shot Expressive Voice Cloning Method Based on VITS – February 2023
Overall structure of the proposed model.
Neural Codec Language Models are Zero-Shot Text to Speech Synthesizers – January 2023
This model is also known as VALL-E and was constructed by Microsoft’s team. Alongside with the paper, the authors published a demonstration of the results achieved in this link. The main highlight of this system is that it can generate high-quality speech with only a 3 seconds recording. This has set a record when it comes to the amount of time required for voice cloning. Also, the quality seems to be outstanding. In addition, VALL-E outperforms state of the art zero-shot systems in terms of speech naturalness and speaker similarity. Another highlight is that it preserves the speaker’s emotion and the acoustic environment of the 3 seconds recording. Following is a system overview of the VALL-E model. It takes as input the text prompt and the 3 seconds recording and outputs the cloned speech.
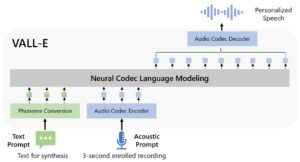
System overview of the proposed voice cloning system.
Low-Resource Multi-lingual and Zero-Shot Multi-speaker TTS – October 2022
In this work, the tasks of zero-shot cloning and multi-lingual low-resource text-to-speech (TTS) are brought together. The authors tried to assess whether the latter can be used to achieve the former in a low-resource scenario. Additionally, they aimed to show that it is possible for a system to learn a new language with just 5 minutes of training data without losing the ability to clone the voices of unseen speakers. This is important because the amount of data needed for previous approaches was generally not feasible for the vast majority of the world’s languages.
To achieve all this, they proposed changes to a TTS encoder to better handle multi-lingual data and disentangle languages from speakers. In addition, they showed that the Language Agnostic Meta Learning (LAML) pre-training procedure can be used to train general speaker-conditioned models. The basic idea of LAML is to treat different languages as separate tasks and iteratively refine an initialization point that is well-suited for all tasks seen in training. This would make it suitable for unseen tasks (i.e. unseen languages or voices in the testing phase). What is important about this paper is that the use of this pre-training procedure could pave the way for future high-quality/low-resource multi-lingual/multi-speaker systems.
Finally, the authors provided a toolkit for teaching, training and using Speech Synthesis models called Toucan.
TorToiSe: An available tool that yields good results
TorToiSe, released in April 2022, is a TTS program that has the ability to imitate voices based on between 2 and 4 given examples. It is made up of 5 neural networks trained independently and then combined to generate the final output. In particular, a combination of Autoregressive Decoders and Denoising Diffusion Probabilistic Models (DDPMs) is used as the authors believe that, when posed with a problem of generating continuous data these models have some distinct advantages. The complete architecture was trained on a dataset with approximately 49k hours of audio which took nearly a year to complete.
The main advantages of this tool are that its results have a good sound quality and it is open-source. Besides, an easy–to–use Jupyter Notebook is provided along with the full implementation. This code allows anyone to use this framework to clone any voice and synthesize speech. However, this notebook can not synthesize long text as the implementation requires a large amount of space. Thus, we used another code to test this framework. This second code can synthesize large texts by splitting it into sentences and concatenating the results for each sentence.
How well does it work?
To begin evaluating the performance of the model we wanted to perform voice cloning for multiple speakers. To this end, we generated a cloned voice for 4 speakers. We first had them record specific sentences generating 4 audio files ranging between 6 and 15 seconds per speaker. Then, these audios were used as input for the system. Finally, we generated multiple texts with the cloned voice. Now we’ll present a comparison between the results and the ground truth.
Text 1: Artificial intelligence has become increasingly important in today’s world because it enables machines to perform tasks that typically require human intelligence.
Overall, the generated audio has very good quality, though at times it lacks expressiveness. On occasion, the audio includes breaths in appropriate places within sentences. However, there are times when the results have improper pauses. This tends to happen when the audios used for cloning contain wrongly placed pauses. Regarding the intonation, it is correct in some cases, but in others, it is a bit flat. The results also show that the English pronunciation of each individual is not cloned. Additionally, the generated audio may include strange noises, as is the case of speaker 2 with text 2.
Text 2: This technology has the potential to revolutionize industries and improve many aspects of our lives, including healthcare, transportation, and education.
There are instances where the cloned voice may sound robotic, as is the case of speaker 2 with text 3. All in all, while the generated audio is not perfect, it allows any user to clone a target voice with little audio and provides good results.
Text 3: The cat slept peacefully on the windowsill, enjoying the warmth of the sun.
Can it generate long sentences?
When generating longer sentences sometimes the result may contain repeated words this happened for speaker 2 with text 4.
Text 4: The color of the sky appears blue during the day because of the way Earth’s atmosphere scatters sunlight. Blue light is scattered more than other colors due to its shorter wavelength, making it more visible to the human eye. As the sun sets or rises, the light must pass through more of the atmosphere, scattering more of the blue light and allowing more reds and oranges to be visible, resulting in a colorful sky.
What about producing text in other languages?
As the model was trained in English, we wanted to test the system’s performance with audio in another language. To this end, we fed it 4 audio files in Spanish and then synthesized other texts in Spanish and English.
Text 5: La luz del sol ilumina suavemente el paisaje, creando sombras y reflejos en las hojas de los árboles y en el agua del río cercano.
Text 6: The color of the sky appears blue during the day because of the way Earth’s atmosphere scatters sunlight. Blue light is scattered more than other colors due to its shorter wavelength, making it more visible to the human eye. As the sun sets or rises, the light must pass through more of the atmosphere, scattering more of the blue light and allowing more reds and oranges to be visible, resulting in a colorful sky.
These results show that TorToiSe can not generate text in other languages. In particular, the generated speech is at times not correctly pronounced and most of the time straight out nonsense. However, when trained with a Spanish speaker it is able to clone the target voice and generate speech in English. From this, we concluded that, even though TorToiSe can not generate text in other languages, it may be used to generate audio with a target voice in a language not spoken by the subject.
How long does it take to generate an audio?
The author reported a 1 to 10 proportion of generated audio duration vs. generation time. To verify this statement we ran multiple tests generating audio with different durations. From this, we discovered that the affirmation is true, the system is quite slow. This makes it unfit for real-time applications and certainly justifies the framework’s name.
Current challenges
Voice cloning technology has made significant progress in recent years, but there are still some technical challenges to be addressed. One major challenge is the ability to generate high-quality synthesized speech that sounds natural and human-like. While current models have shown promising results, there is still room for improvement.
Further challenges include the need for large amounts of training data to develop accurate and reliable voice clones. Voice cloning models require large datasets (thousands of hours) of high-quality recordings in order to learn the unique characteristics of a person’s voice. However, obtaining such datasets can be time-consuming and expensive, especially to clone individuals with no available data.
Another challenge in the field is the issue of speaker variability. Voice cloning models are typically trained on a single speaker’s voice. This can limit their ability to accurately clone other speakers with different speech patterns and accents. To address this, researchers are exploring techniques such as multi-speaker training and domain adaptation.
Lastly, the issue of real-time voice cloning remains a challenge. While current models can generate high-quality synthesized speech, they are not yet capable of producing it in real-time. This limits their potential use cases in applications such as voice assistants and chatbots. Addressing these technical challenges will be crucial for the continued advancement and widespread adoption of this technology.
Other voice cloning alternatives
To save you some time, here are other resources not mentioned in this article that you may stumble upon.
Open-Source alternatives
- PaddleSpeech: Open-source toolkit on the PaddlePaddle platform for a variety of critical tasks in speech and audio. In the library’s GitHub we found the code provided is not end to end.
- Coqui TTS: It is described as a library for advanced text-to-speech generation. Nonetheless, the resulting audios tend to sound noisy, with unnatural breaks in the voice and a metallic ring. Click here to find the authors demo.
- HuggingFace: Multiple voice cloning demos can be found, by different authors. This implementations do not come close to the performance reached by TorToiSe. Some examples can be found in the following links: Link 1, Link 2.
Private implementations
- Self-supervised learning for robust voice cloning: This paper presents an algorithm that can be trained on an unlabeled dataset with any number of speakers. The results presented are close to the baseline performance of speaker features pre-trained on speaker verification tasks. The authors have not provided any implementation to this date.
- Zero-Shot Long-Form Voice Cloning with Dynamic Convolution Attention: The model proposed in this paper is based on Tacotron 2 and uses an energy based attention mechanism (Dynamic Convolution Attention) combined with a set of modifications for Tacotron 2’s synthesizer. The authors concluded that the proposed model can produce intelligible synthetic speech, while preserving naturalness and similarity. We found no code for this framework during the course of this research.
- NVIDIA Riva Studio: Allows you to clone voices using only 30 minutes of audio recordings with a no-code approach. However, this tool is still in an early access stage and its use is limited to developers working on text-to-speech.
Paid services
- Resemble AI: Their voice generator claims to generate human-like voice overs in seconds and lets you clone your voice for free. Keep in mind that it took more than 3 weeks for them to generate one voice using the free trial. The process of voice cloning is based initially on 25 audio recordings of the target voice with predefined text. They also provide a paid alternative that allows users to better the cloned voice by adding more audio samples. In addition, there is a recently added Rapid Voice Cloning framework from just 10 seconds of reference audio. In our experience, this provided flatly intonated audio with little resemblance to the original voice.
- Custom Neural Voice Lite: Tool by Microsoft Azure that enables users to clone voices from 5 minutes of recorded speech. It has three main use requirements. First you must have a Microsoft Azure subscription. Also, you must fill out an application and it has to be approved, this step can take up to 10 days. Finally, you must attain to the user guidelines proposed by Microsoft’s team.
- Cloud Text-to-Speech API: This is a service that offers Custom Voices. It allows you to train a custom voice model using your own audio recordings to create a unique voice. You can then use your custom voice to synthesize audio using the API. Keep in mind that this is a paid service but you can try it by enrolling for a free trial.
Final thoughts
Wrapping up, the voice cloning technology has come a long way and is on its way to revolutionize various industries. On the one hand, we saw that the latest advancements include the zero-shot expressive voice cloning method based on VITS, VALL-E, and the Low-Resource Zero-Shot Multi-speaker TTS.
Also, we identified ethical considerations related to consent and identity which must be addressed to prevent the misuse of this technology. Additionally, we delve into the best open source voice cloning tool available, analyzing both its strengths and weaknesses. TorToiSe is a tool that is readily available and yields good results.
To conclude, all these recent breakthroughs demonstrate the potential of voice cloning to create high-quality personalized speech with minimal data, thus it is easy to see that this technology is on the fast-track to success.